In this tutorial, you will learn how to train your own custom dlib shape predictor. You’ll then learn how to take your trained dlib shape predictor and use it to predict landmarks on input images and real-time video streams.
Today kicks off a brand new two-part series on training custom shape predictors with dlib:
- Part #1: Training a custom dlib shape predictor (today’s tutorial)
- Part #2: Tuning dlib shape predictor hyperparameters to balance speed, accuracy, and model size (next week’s tutorial)
Shape predictors, also called landmark predictors, are used to predict key (x, y)-coordinates of a given “shape”.
The most common, well-known shape predictor is dlib’s facial landmark predictor used to localize individual facial structures, including the:
- Eyes
- Eyebrows
- Nose
- Lips/mouth
- Jawline
Facial landmarks are used for face alignment (a method to improve face recognition accuracy), building a “drowsiness detector” to detect tired, sleepy drivers behind the wheel, face swapping, virtual makeover applications, and much more.
However, just because facial landmarks are the most popular type of shape predictor, doesn’t mean we can’t train a shape predictor to localize other shapes in an image!
For example, you could use a shape predictor to:
- Automatically localize the four corners of a piece of paper when building a computer vision-based document scanner.
- Detect the key, structural joints of the human body (feet, knees, elbows, etc.).
- Localize the tips of your fingers when building an AR/VR application.
Today we’ll be exploring shape predictors in more detail, including how you can train your own custom shape predictor using the dlib library.
To learn how to train your own dlib shape predictor, just keep reading!
Looking for the source code to this post?
Jump right to the downloads section.
Tuning a custom dlib shape predictor
In the first part of this tutorial, we’ll briefly discuss what shape/landmark predictors are and how they can be used to predict specific locations on structural objects.
From there we’ll review the iBUG 300-W dataset, a common dataset used to train shape predictors used to localize specific locations on the human face (i.e., facial landmarks).
I’ll then show you how to train your own custom dlib shape predictor, resulting in a model that can balance speed, accuracy, and model size.
Finally, we’ll put our shape predictor to the test and apply it to a set of input images/video streams, demonstrating that our shape predictor is capable of running in real-time.
We’ll wrap up the tutorial with a discussion of next steps.
What are shape/landmark predictors?
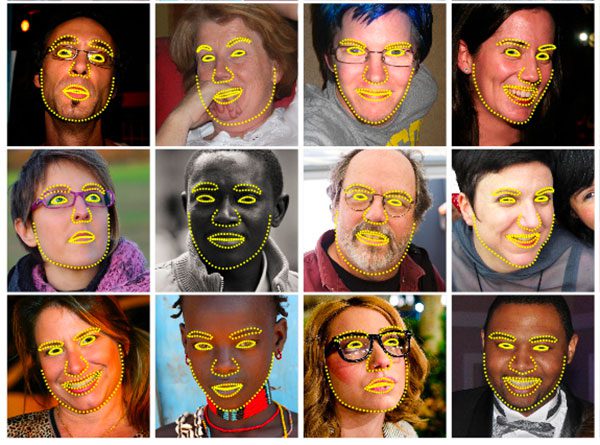
Figure 1: Training a custom dlib shape predictor on facial landmarks (image source).
Shape/landmark predictors are used to localize specific (x, y)-coordinates on an input “shape”. The term “shape” is is arbitrary, but it’s assumed that the shape is structural in nature.
Examples of structural shapes include:
- Faces
- Hands
- Fingers
- Toes
- etc.
For example, faces come in all different shapes and sizes, and they all share common structural characteristics — the eyes are above the nose, the nose is above the mouth, etc.
The goal of shape/landmark predictors is to exploit this structural knowledgem and given enough training data, learn how to automatically predict the location of these structures.
How do shape/landmark predictors work?
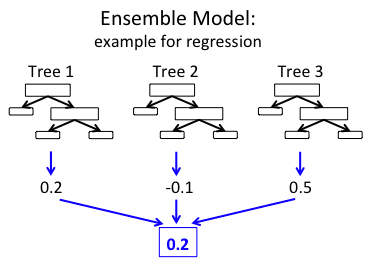
Figure 2: How do shape/landmark predictors work? The dlib library implements a shape predictor algorithm with an ensemble of regression tress approach using the method described by Kazemi and Sullivan in their 2014 CVPR paper (image source).
There are a variety of shape predictor algorithms. Exactly which one you use depends on whether:
- You’re working with 2D or 3D data
- You need to utilize deep learning
- Or, if traditional Computer Vision and Machine Learning algorithms will suffice
The shape predictor algorithm implemented in the dlib library comes from Kazemi and Sullivan 2014 CVPR paper, One Millisecond Face Alignment with an Ensemble of Regression Trees.
To estimate the landmark locations, the algorithm:
- Examines a sparse set of input pixel intensities (i.e., the “features” to the input model)
- Passes the features into an Ensemble of Regression Trees (ERT)
- Refines the predicted locations to improve accuracy through a cascade of regressors
The end result is a shape predictor that can run in super real-time!
For more details on the inner-workings of the landmark prediction, be sure to refer to Kazemi and Sullivan’s 2014 publication.
The iBUG 300-W dataset
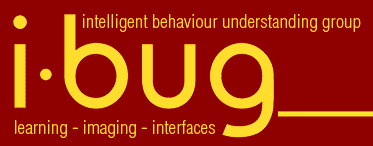
Figure 3: In this tutorial we will use the iBUG 300-W face landmark dataset to learn how to train a custom dlib shape predictor.
To train our custom dlib shape predictor, we’ll be utilizing the iBUG 300-W dataset (but with a twist).
The goal of iBUG-300W is to train a shape predictor capable of localizing each individual facial structure, including the eyes, eyebrows, nose, mouth, and jawline.
The dataset itself consists of 68 pairs of integer values — these values are the (x, y)-coordinates of the facial structures are depicted in Figure 2 above.
To create the iBUG-300W dataset, researchers manually and painstakingly annotated and labeled each of the 68 coordinates on a total of 7,764 images.
A model trained on iBUG-300W can predict the location of each of these 68 (x, y)-coordinate pairs and can, therefore, localize each of the locations on the face.
That’s all fine and good…
…but what if wanted to train a shape predictor to localize just the eyes?
How might we go about doing that?
Balancing shape predictor model speed and accuracy
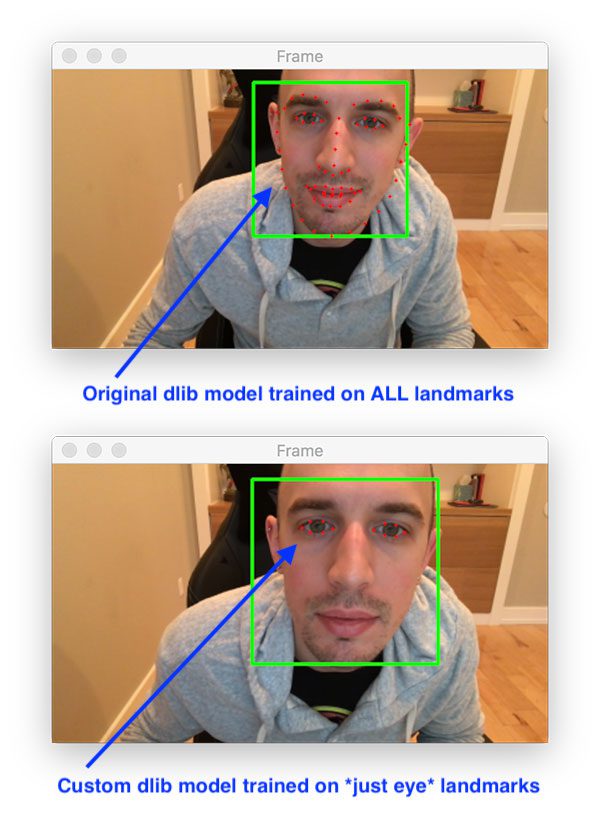
Figure 4: We will train a custom dlib shape/landmark predictor to recognize just eyes in this tutorial.
Let’s suppose for a second that you want to train a custom shape predictor to localize just the location of the eyes.
We would have two options to accomplish this task:
- Utilize dlib’s pre-trained facial landmark detector used to localize all facial structures and then discard all localizations except for the eyes.
- Train our own custom dlib landmark predictor that returns just the locations of the eyes.
In some cases you may be able to get away with the first option; however, there are two problems there, namely regarding your model speed and your model size.
Model speed: Even though you’re only interested in a subset of the landmark predictions, your model is still responsible for predicting the entire set of landmarks. You can’t just tell your model “Oh hey, just give me those locations, don’t bother computing the rest.” It doesn’t work like that — it’s an “all or nothing” calculation.
Model size: Since your model needs to know how to predict all landmark locations it was trained on, it therefore needs to store quantified information on how to predict each of these locations. The more information it needs to store, the larger your model size is.
Think of your shape predictor model size as a grocery list — out of a list of 20 items, you may only truly need eggs and a gallon of milk, but if you’re heading to the store, you’re going to be purchasing all the items on that list because that’s what your family expects you to do!
The model size is the same way.
Your model doesn’t “care” that you only truly “need” a subset of the landmark predictions; it was trained to predict all of them so you’re going to get all of them in return!
If you only need a subset of specific landmarks you should consider training your own custom shape predictor — you’ll end up with a model that is both smaller and faster.
In the context of today’s tutorial, we’ll be training a custom dlib shape predictor to localize just the eye locations from the iBUG 300-W dataset.
Such a model could be utilized in a virtual makeover application used to apply just eyeliner/mascara or it could be used in a drowsiness detector used to detect tired drivers behind the wheel of a car.
Configuring your dlib development environment
To follow along with today’s tutorial, you will need a virtual environment with the following packages installed:
- dlib
- OpenCV
- imutils
Luckily, each of these packages is pip-installable, but there are a handful of pre-requisites including virtual environments. Be sure to follow these two guides for additional information:
The pip install commands include:
$ workon <env-name> $ pip install dlib $ pip install opencv-contrib-python $ pip install imutils
The
workoncommand becomes available once you install
virtualenvand
virtualenvwrapperper either my dlib or OpenCV installation guides.
Downloading the iBUG 300-W dataset
Before we get too far into this tutorial, take a second now to download the iBUG 300-W dataset (~1.7GB):
http://dlib.net/files/data/ibug_300W_large_face_landmark_dataset.tar.gz
You’ll also want to use the “Downloads” section of this blog post to download the source code.
I recommend placing the iBug 300W dataset into the zip associated with the download of this tutorial like this:
$ unzip custom-dlib-shape-predictor.zip ... $ cd custom-dlib-shape-predictor $ mv ~/Downloads/ibug_300W_large_face_landmark_dataset.tar.gz . $ tar -xvf ibug_300W_large_face_landmark_dataset.tar.gz ...
Alternatively (i.e. rather than clicking the hyperlink above), use
wgetin your terminal to download the dataset directly:
$ unzip custom-dlib-shape-predictor.zip ... $ cd custom-dlib-shape-predictor $ wget http://dlib.net/files/data/ibug_300W_large_face_landmark_dataset.tar.gz $ tar -xvf ibug_300W_large_face_landmark_dataset.tar.gz ...
From there you can follow along with the rest of the tutorial.
Project Structure
Assuming you have followed the instructions in the previous section, your project directory is now organized as follows:
$ tree --dirsfirst --filelimit 10 . ├── ibug_300W_large_face_landmark_dataset │ ├── afw [1011 entries] │ ├── helen │ │ ├── testset [990 entries] │ │ └── trainset [6000 entries] │ ├── ibug [405 entries] │ ├── image_metadata_stylesheet.xsl │ ├── labels_ibug_300W.xml │ ├── labels_ibug_300W_test.xml │ ├── labels_ibug_300W_train.xml │ └── lfpw │ ├── testset [672 entries] │ └── trainset [2433 entries] ├── ibug_300W_large_face_landmark_dataset.tar.gz ├── eye_predictor.dat ├── parse_xml.py ├── train_shape_predictor.py ├── evaluate_shape_predictor.py └── predict_eyes.py 9 directories, 10 files
The iBug 300-W dataset is extracted in the
ibug_300W_large_face_landmark_dataset/directory. We will review the following Python scripts in this order:
parse_xml.py
: Parses the train/test XML dataset files for eyes-only landmark coordinates.train_shape_predictor.py
: Accepts the parsed XML files to train our shape predictor with dlib.evaluate_shape_predictor.py
: Calculates the Mean Average Error (MAE) of our custom shape predictor.predict_eyes.py
: Performs shape prediction using our custom dlib shape predictor, trained to only recognize eye landmarks.
We’ll begin by inspecting our input XML files in the next section.
Understanding the iBUG-300W XML file structure
We’ll be using the iBUG-300W to train our shape predictor; however, we have a bit of a problem:
iBUG-300W supplies (x, y)-coordinate pairs for all facial structures in the dataset (i.e., eyebrows, eyes, nose, mouth, and jawline)…
…however, we want to train our shape predictor on just the eyes!
So, what are we going to do?
Are we going to find another dataset that doesn’t include the facial structures we don’t care about?
Manually open up the training file and delete the coordinate pairs for the facial structures we don’t need?
Simply give up, take our ball, and go home?
Of course not!
We’re programmers and engineers — all we need is some basic file parsing to create a new training file that includes just the eye coordinates.
To understand how we can do that, let’s first consider how facial landmarks are annotated in the iBUG-300W dataset by examining the
labels_ibug_300W_train.xmltraining file:
... <images> <image file='lfpw/trainset/image_0457.png'> <box top='78' left='74' width='138' height='140'> <part name='00' x='55' y='141'/> <part name='01' x='59' y='161'/> <part name='02' x='66' y='182'/> <part name='03' x='75' y='197'/> <part name='04' x='90' y='209'/> <part name='05' x='108' y='220'/> <part name='06' x='131' y='226'/> <part name='07' x='149' y='232'/> <part name='08' x='167' y='230'/> <part name='09' x='181' y='225'/> <part name='10' x='184' y='208'/> <part name='11' x='186' y='193'/> <part name='12' x='185' y='179'/> <part name='13' x='184' y='167'/> <part name='14' x='186' y='152'/> <part name='15' x='185' y='142'/> <part name='16' x='181' y='133'/> <part name='17' x='95' y='128'/> <part name='18' x='105' y='121'/> <part name='19' x='117' y='117'/> <part name='20' x='128' y='115'/> <part name='21' x='141' y='116'/> <part name='22' x='156' y='115'/> <part name='23' x='162' y='110'/> <part name='24' x='169' y='108'/> <part name='25' x='175' y='108'/> <part name='26' x='180' y='109'/> <part name='27' x='152' y='127'/> <part name='28' x='157' y='136'/> <part name='29' x='162' y='145'/> <part name='30' x='168' y='154'/> <part name='31' x='152' y='166'/> <part name='32' x='158' y='166'/> <part name='33' x='163' y='168'/> <part name='34' x='167' y='166'/> <part name='35' x='171' y='164'/> <part name='36' x='111' y='134'/> <part name='37' x='116' y='130'/> <part name='38' x='124' y='128'/> <part name='39' x='129' y='130'/> <part name='40' x='125' y='134'/> <part name='41' x='118' y='136'/> <part name='42' x='161' y='127'/> <part name='43' x='166' y='123'/> <part name='44' x='173' y='122'/> <part name='45' x='176' y='125'/> <part name='46' x='173' y='129'/> <part name='47' x='167' y='129'/> <part name='48' x='139' y='194'/> <part name='49' x='151' y='186'/> <part name='50' x='159' y='180'/> <part name='51' x='163' y='182'/> <part name='52' x='168' y='180'/> <part name='53' x='173' y='183'/> <part name='54' x='176' y='189'/> <part name='55' x='174' y='193'/> <part name='56' x='170' y='197'/> <part name='57' x='165' y='199'/> <part name='58' x='160' y='199'/> <part name='59' x='152' y='198'/> <part name='60' x='143' y='194'/> <part name='61' x='159' y='186'/> <part name='62' x='163' y='187'/> <part name='63' x='168' y='186'/> <part name='64' x='174' y='189'/> <part name='65' x='168' y='191'/> <part name='66' x='164' y='192'/> <part name='67' x='160' y='192'/> </box> </image> ...
All training data in the iBUG-300W dataset is represented by a structured XML file.
Each image has an
imagetag.
Inside the
imagetag is a
fileattribute that points to where the example image file resides on disk.
Additionally, each
imagehas a
boxelement associated with it.
The
boxelement represents the bounding box coordinates of the face in the image. To understand how the
boxelement represents the bounding box of the face, consider its four attributes:
top
: The starting y-coordinate of the bounding box.left
: The starting x-coordinate of the bounding box.width
: The width of the bounding box.height
: The height of the bounding box.
Inside the
boxelement we have a total of 68
partelements — these
partelements represent the individual (x, y)-coordinates of the facial landmarks in the iBUG-300W dataset.
Notice that each
partelement has three attributes:
name
: The index/name of the specific facial landmark.x
: The x-coordinate of the landmark.y
: The y-coordinate of the landmark.
So, how do these landmarks map to specific facial structures?
The answer lies in the following figure:
The coordinates in Figure 5 are 1-indexed so to map the coordinate
nameto our XML file, simply subtract 1 from the value (since our XML file is 0-indexed).
Based on the visualization, we can then derive which
namecoordinates maps to which facial structure:
- The mouth can be accessed through points [48, 68].
- The right eyebrow through points [17, 22].
- The left eyebrow through points [22, 27].
- The right eye using [36, 42].
- The left eye with [42, 48].
- The nose using [27, 35].
- And the jaw via [0, 17].
Since we’re only interested in the eyes, we therefore need to parse out points [36, 48), again keeping in mind that:
- Our coordinates are zero-indexed in the XML file
- And the closing parenthesis “)” in [36, 48) is mathematical notation implying “non-inclusive”.
Now that we understand the structure of the iBUG-300W training file, we can move on to parsing out only the eye coordinates.
Building an “eyes only” shape predictor dataset
Let’s create a Python script to parse the iBUG-300W XML files and extract only the eye coordinates (which we’ll then train a custom dlib shape predictor on in the following section).
Open up the
parse_xml.pyfile and we’ll get started:
# import the necessary packages import argparse import re # construct the argument parser and parse the arguments ap = argparse.ArgumentParser() ap.add_argument("-i", "--input", required=True, help="path to iBug 300-W data split XML file") ap.add_argument("-t", "--output", required=True, help="path output data split XML file") args = vars(ap.parse_args())
Lines 2 and 3 import necessary packages.
We’ll use two of Python’s built-in modules: (1)
argparsefor parsing command line arguments, and (2)
refor regular expression matching. If you ever need help developing regular expressions, regex101.com is a great tool and supports languages other than Python as well.
Our script requires two command line arguments:
--input
: The path to our input data split XML file (i.e. from the iBug 300-W dataset).--output
: The path to our output eyes-only output XML file.
Let’s go ahead and define the indices of our eye coordinates:
# in the iBUG 300-W dataset, each (x, y)-coordinate maps to a specific # facial feature (i.e., eye, mouth, nose, etc.) -- in order to train a # dlib shape predictor on *just* the eyes, we must first define the # integer indexes that belong to the eyes LANDMARKS = set(list(range(36, 48)))
Our eye landmarks are specified on Line 17. Refer to Figure 5, keeping in mind that the figure is 1-indexed while Python is 0-indexed.
We’ll be training our custom shape predictor on eye locations; however, you could just as easily train an eyebrow, nose, mouth, or jawline predictor, including any combination or subset of these structures, by modifying the
LANDMARKSlist and including the 0-indexed names of the landmarks you want to detect.
Now let’s define our regular expression and load the original input XML file:
# to easily parse out the eye locations from the XML file we can # utilize regular expressions to determine if there is a 'part' # element on any given line PART = re.compile("part name='[0-9]+'") # load the contents of the original XML file and open the output file # for writing print("[INFO] parsing data split XML file...") rows = open(args["input"]).read().strip().split("\n") output = open(args["output"], "w")
Our regular expression on Line 22 will soon enable extracting
partelements along with their names/indexes.
Line 27 loads the contents of input XML file.
Line 28 opens our output XML file for writing.
Now we’re ready to loop over the input XML file to find and extract the eye landmarks:
# loop over the rows of the data split file for row in rows: # check to see if the current line has the (x, y)-coordinates for # the facial landmarks we are interested in parts = re.findall(PART, row) # if there is no information related to the (x, y)-coordinates of # the facial landmarks, we can write the current line out to disk # with no further modifications if len(parts) == 0: output.write("{}\n".format(row)) # otherwise, there is annotation information that we must process else: # parse out the name of the attribute from the row attr = "name='" i = row.find(attr) j = row.find("'", i + len(attr) + 1) name = int(row[i + len(attr):j]) # if the facial landmark name exists within the range of our # indexes, write it to our output file if name in LANDMARKS: output.write("{}\n".format(row)) # close the output file output.close()
Line 31 begins a loop over the
rowsof the input XML file. Inside the loop, we perform the following tasks:
- Determine if the current
row
contains apart
element via regular expression matching (Line 34).- If it does not contain a
part
element, write the row back out to file (Lines 39 and 40). - If it does contain a
part
element, we need to parse it further (Lines 43-53).- Here we extract
name
attribute from thepart
. - And then check to see if the
name
exists in theLANDMARKS
we want to train a shape predictor to localize. If so, we write therow
back out to disk (otherwise we ignore the particularname
as it’s not a landmark we want to localize).
- Here we extract
- If it does not contain a
- Wrap up the script by closing our
output
XML file (Line 56).
Note: Most of our parse_xml.py
script was inspired by Luca Anzalone’s slice_xml
function from their GitHub repo. A big thank you to Luca for putting together such a simple, concise script that is highly effective!
Creating our training and testing splits
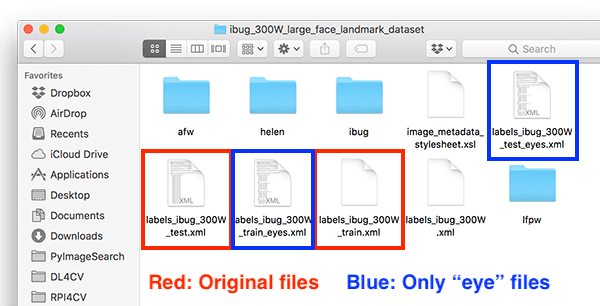
Figure 6: Creating our “eye only” face landmark training/testing XML files for training a dlib custom shape predictor with Python.
At this point in the tutorial I assume you have both:
- Downloaded the iBUG-300W dataset from the “Downloading the iBUG 300-W dataset” section above
- Used the “Downloads” section of this tutorial to download the source code.
You can use the following command to generate our new training file by parsing only the eye landmark coordinates from the original training file:
$ python parse_xml.py \ --input ibug_300W_large_face_landmark_dataset/labels_ibug_300W_train.xml \ --output ibug_300W_large_face_landmark_dataset/labels_ibug_300W_train_eyes.xml [INFO] parsing data split XML file...
Similarly, you can do the same to create our new testing file:
$ python parse_xml.py \ --input ibug_300W_large_face_landmark_dataset/labels_ibug_300W_test.xml \ --output ibug_300W_large_face_landmark_dataset/labels_ibug_300W_test_eyes.xml [INFO] parsing data split XML file...
To verify that our new training/testing files have been created, check your iBUG-300W root dataset directory for the
labels_ibug_300W_train_eyes.xmland
labels_ibug_300W_test_eyes.xmlfiles:
$ cd ibug_300W_large_face_landmark_dataset $ ls -lh *.xml -rw-r--r--@ 1 adrian staff 21M Aug 16 2014 labels_ibug_300W.xml -rw-r--r--@ 1 adrian staff 2.8M Aug 16 2014 labels_ibug_300W_test.xml -rw-r--r-- 1 adrian staff 602K Dec 12 12:54 labels_ibug_300W_test_eyes.xml -rw-r--r--@ 1 adrian staff 18M Aug 16 2014 labels_ibug_300W_train.xml -rw-r--r-- 1 adrian staff 3.9M Dec 12 12:54 labels_ibug_300W_train_eyes.xml $ cd ..
Notice that our
*_eyes.xmlfiles are highlighted. Both of these files are significantly smaller in filesize than their original, non-parsed counterparts.
Implementing our custom dlib shape predictor training script
Our dlib shape predictor training script is loosely based on (1) dlib’s official example and (2) Luca Anzalone’s excellent 2018 article.
My primary contributions here are to:
- Supply a complete end-to-end example of creating a custom dlib shape predictor, including:
- Training the shape predictor on a training set
- Evaluating the shape predictor on a testing set
- Use the shape predictor to make predictions on custom images/video streams.
- Provide additional commentary on the hyperparameters you should be tuning.
- Demonstrate how to systematically tune your shape predictor hyperparameters to balance speed, model size, and accuracy (next week’s tutorial).
To learn how to train your own dlib shape predictor, open up the
train_shape_predictor.pyfile in your project structure and insert the following code:
# import the necessary packages import multiprocessing import argparse import dlib # construct the argument parser and parse the arguments ap = argparse.ArgumentParser() ap.add_argument("-t", "--training", required=True, help="path to input training XML file") ap.add_argument("-m", "--model", required=True, help="path serialized dlib shape predictor model") args = vars(ap.parse_args())
Lines 2-4 import our packages, namely dlib. The dlib toolkit is a package developed by PyImageConf 2018 speaker, Davis King. We will use dlib to train our shape predictor.
The multiprocessing library will be used to grab and set the number of threads/processes we will use for training our shape predictor.
Our script requires two command line arguments (Lines 7-12):
--training
: The path to our input training XML file. We will use the eyes-only XML file generated by the previous two sections.--model
: The path to the serialized dlib shape predictor output file.
From here we need to set options (i.e., hyperparameters) prior to training the shape predictor.
While the following code blocks could be condensed into just 11 lines of code, the comments in both the code and in this tutorial provide additional information to help you both (1) understand the key options, and (2) configure and tune the options/hyperparameters for optimal performance.
In the remaining code blocks in this section I’ll be discussing the 7 most important hyperparameters you can tune/set when training your own custom dlib shape predictor. These values are:
tree_depth
nu
cascade_depth
feature_pool_size
num_test_splits
oversampling_amount
oversampling_translation_jitter
We’ll begin with grabbing the default dlib shape predictor options:
# grab the default options for dlib's shape predictor print("[INFO] setting shape predictor options...") options = dlib.shape_predictor_training_options()
From there, we’ll configure the
tree_depthoption:
# define the depth of each regression tree -- there will be a total # of 2^tree_depth leaves in each tree; small values of tree_depth # will be *faster* but *less accurate* while larger values will # generate trees that are *deeper*, *more accurate*, but will run # *far slower* when making predictions options.tree_depth = 4
Here we define the
tree_depth, which, as the name suggests, controls the depth of each regression tree in the Ensemble of Regression Trees (ERTs). There will be
2^tree_depthleaves in each tree — you must be careful to balance depth with speed.
Smaller values of
tree_depthwill lead to more shallow trees that are faster, but potentially less accurate. Larger values of
tree_depthwill create deeper trees that are slower, but potentially more accurate.
Typical values for
tree_depthare in the range [2, 8].
The next parameter we’re going to explore is
nu, a regularization parameter:
# regularization parameter in the range [0, 1] that is used to help # our model generalize -- values closer to 1 will make our model fit # the training data better, but could cause overfitting; values closer # to 0 will help our model generalize but will require us to have # training data in the order of 1000s of data points options.nu = 0.1
The
nuoption is a floating-point value (in the range [0, 1]) used as a regularization parameter to help our model generalize.
Values closer to
1will make our model fit the training data closer, but could potentially lead to overfitting. Values closer to
0will help our model generalize; however, there is a caveat to the generalization power — the closer
nuis to
0, the more training data you’ll need.
Typically, for small values of
nuyou’ll need 1000s of training examples.
Our next parameter is the
cascade_depth:
# the number of cascades used to train the shape predictor -- this # parameter has a *dramtic* impact on both the *accuracy* and *output # size* of your model; the more cascades you have, the more accurate # your model can potentially be, but also the *larger* the output size options.cascade_depth = 15
A series of cascades are used to refine and tune the initial predictions from the ERTs — the cascade_depth
will have a dramatic impact on both the accuracy and the output file size of your model.
The more cascades you allow for, the larger your model will become (but potentially more accurate). The fewer cascades you allow, the smaller your model will be (but could be less accurate).
The following figure from Kazemi and Sullivan’s paper demonstrates the impact that the
cascade_depthhas on facial landmark alignment:
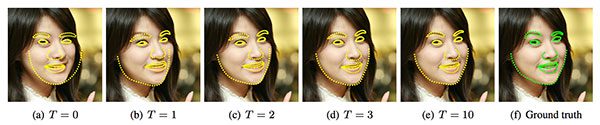
Figure 7: The cascade_depth
parameter has a significant impact on the accuracy of your custom dlib shape/landmark predictor model.
Clearly you can see that the deeper the cascade, the better the facial landmark alignment.
Typically you’ll want to explore
cascade_depthvalues in the range [6, 18], depending on your required target model size and accuracy.
Let’s now move on to the
feature_pool_size:
# number of pixels used to generate features for the random trees at # each cascade -- larger pixel values will make your shape predictor # more accurate, but slower; use large values if speed is not a # problem, otherwise smaller values for resource constrained/embedded # devices options.feature_pool_size = 400
The
feature_pool_sizecontrols the number of pixels used to generate features for the random trees in each cascade.
The more pixels you include, the slower your model will run (but could potentially be more accurate). The fewer pixels you take into account, the faster your model will run (but could also be less accurate).
My recommendation here is that you should use large values for feature_pools_size
if inference speed is not a concern. Otherwise, you should use smaller values for faster prediction speed (typically for embedded/resource-constrained devices).
The next parameter we’re going to set is the
num_test_splits:
# selects best features at each cascade when training -- the larger # this value is, the *longer* it will take to train but (potentially) # the more *accurate* your model will be options.num_test_splits = 50
The
num_test_splitsparameter has a dramatic impact on how long it takes your model to train (i.e., training/wall clock time, not inference speed).
The more
num_test_splitsyou consider, the more likely you’ll have an accurate shape predictor — but again, take caution will this parameter as it can cause training time to explode.
Let’s check out the
oversampling_amountnext:
# controls amount of "jitter" (i.e., data augmentation) when training # the shape predictor -- applies the supplied number of random # deformations, thereby performing regularization and increasing the # ability of our model to generalize options.oversampling_amount = 5
The
oversampling_amountcontrols the amount of data augmentation applied to our training data. The dlib library causes data augmentation jitter, but it is essentially the same idea as data augmentation.
Here we are telling dlib to apply a total of
5random deformations to each input image.
You can think of the
oversampling_amountas a regularization parameter as it may lower training accuracy but increase testing accuracy, thereby allowing our model to generalize better.
Typical
oversampling_amountvalues lie in the range [0, 50] where
0means no augmentation and
50is a 50x increase in your training dataset.
Be careful with this parameter! Larger
oversampling_amountvalues may seem like a good idea but it can dramatically increase your training time.
Next comes the
oversampling_translation_jitteroption:
# amount of translation jitter to apply -- the dlib docs recommend # values in the range [0, 0.5] options.oversampling_translation_jitter = 0.1
The
oversampling_translation_jittercontrols the amount of translation augmentation applied to our training dataset.
Typical values for translation jitter lie in the range [0, 0.5].
The
be_verboseoption simply instructs dlib to print out status messages as our shape predictor is training:
# tell the dlib shape predictor to be verbose and print out status # messages our model trains options.be_verbose = True
Finally, we have the
num_threadsparameter:
# number of threads/CPU cores to be used when training -- we default # this value to the number of available cores on the system, but you # can supply an integer value here if you would like options.num_threads = multiprocessing.cpu_count()
This parameter is extremely important as it can dramatically speed up the time it takes to train your model!
The more CPU threads/cores you can supply to dlib, the faster your model will train. We’ll default this value to the total number of CPUs on our system; however, you can set this value as any integer (provided it’s less-than-or-equal-to the number of CPUs on your system).
Now that our
optionsare set, the final step is to simply call
train_shape_predictor:
# log our training options to the terminal print("[INFO] shape predictor options:") print(options) # train the shape predictor print("[INFO] training shape predictor...") dlib.train_shape_predictor(args["training"], args["model"], options)
The dlib library accepts (1) the path to our training XML file, (2) the path to our output shape predictor model, and (3) our set of options.
Once trained the shape predictor will be serialized to disk so we can later use it.
While this script may have appeared especially easy, be sure to spend time configuring your options/hyperparameters for optimal performance.
Training the custom dlib shape predictor
We are now ready to train our custom dlib shape predictor!
Make sure you have (1) downloaded the iBUG-300W dataset and (2) used the “Downloads” section of this tutorial to download the source code to this post.
Once you have done so, you are ready to train the shape predictor:
$ python train_shape_predictor.py \ --training ibug_300W_large_face_landmark_dataset/labels_ibug_300W_train_eyes.xml \ --model eye_predictor.dat [INFO] setting shape predictor options... [INFO] shape predictor options: shape_predictor_training_options(be_verbose=1, cascade_depth=15, tree_depth=4, num_trees_per_cascade_level=500, nu=0.1, oversampling_amount=5, oversampling_translation_jitter=0.1, feature_pool_size=400, lambda_param=0.1, num_test_splits=50, feature_pool_region_padding=0, random_seed=, num_threads=20, landmark_relative_padding_mode=1) [INFO] training shape predictor... Training with cascade depth: 15 Training with tree depth: 4 Training with 500 trees per cascade level. Training with nu: 0.1 Training with random seed: Training with oversampling amount: 5 Training with oversampling translation jitter: 0.1 Training with landmark_relative_padding_mode: 1 Training with feature pool size: 400 Training with feature pool region padding: 0 Training with 20 threads. Training with lambda_param: 0.1 Training with 50 split tests. Fitting trees... Training complete Training complete, saved predictor to file eye_predictor.dat
The entire training process took 9m11s on my 3 GHz Intel Xeon W processor.
To verify that your shape predictor has been serialized to disk, ensure that
eye_predictor.dathas been created in your directory structure:
$ ls -lh *.dat -rw-r--r--@ 1 adrian staff 18M Dec 4 17:15 eye_predictor.dat
As you can see, the output model is only 18MB — that’s quite the reduction in file size compared to dlib’s standard/default facial landmark predictor which is 99.7MB!
Implementing our shape predictor evaluation script
Now that we’ve trained our dlib shape predictor, we need to evaluate its performance on both our training and testing sets to verify that it’s not overfitting and that our results will (ideally) generalize to our own images outside the training set.
Open up the
evaluate_shape_predictor.pyfile and insert the following code:
# import the necessary packages import argparse import dlib # construct the argument parser and parse the arguments ap = argparse.ArgumentParser() ap.add_argument("-p", "--predictor", required=True, help="path to trained dlib shape predictor model") ap.add_argument("-x", "--xml", required=True, help="path to input training/testing XML file") args = vars(ap.parse_args()) # compute the error over the supplied data split and display it to # our screen print("[INFO] evaluating shape predictor...") error = dlib.test_shape_predictor(args["xml"], args["predictor"]) print("[INFO] error: {}".format(error))
Lines 2 and 3 indicate that we need both
argparseand
dlibto evaluate our shape predictor.
Our command line arguments include:
--predictor
: The path to our serialized shape predictor model that we generated via the previous two “Training” sections.--xml
: The path to the input training/testing XML file (i.e. our eyes-only parsed XML files).
When both of these arguments are provided via the command line, dlib will handle evaluation (Line 16). Dlib handles computing the mean average error (MAE) between the predicted landmark coordinates and the ground-truth landmark coordinates.
The smaller the MAE, the better the predictions.
Shape prediction accuracy results
If you haven’t yet, use the “Downloads” section of this tutorial to download the source code and pre-trained shape predictor.
From there, execute the following command to evaluate our eye landmark predictor on the training set:
$ python evaluate_shape_predictor.py --predictor eye_predictor.dat \ --xml ibug_300W_large_face_landmark_dataset/labels_ibug_300W_train_eyes.xml [INFO] evaluating shape predictor... [INFO] error: 3.631152776257545
Here we are obtaining an MAE of ~3.63.
Let’s now run the same command on our testing set:
$ python evaluate_shape_predictor.py --predictor eye_predictor.dat \ --xml ibug_300W_large_face_landmark_dataset/labels_ibug_300W_test_eyes.xml [INFO] evaluating shape predictor... [INFO] error: 7.568211111799696
As you can see the MAE is twice as large on our testing set versus our training set.
If you have any prior experience working with machine learning or deep learning algorithms you know that in most situations, your training loss will be lower than your testing loss. That doesn’t mean that your model is performing badly — instead, it simply means that your model is doing a better job modeling the training data versus the testing data.
Shape predictors are especially interesting to evaluate as it’s not just the MAE that needs to be examined!
You also need to visually validate the results and verify the shape predictor is working as expected — we’ll cover that topic in the next section.
Implementing the shape predictor inference script
Now that we have our shape predictor trained, we need to visually validate that the results look good by applying it to our own example images/video.
In this section we will:
- Load our trained dlib shape predictor from disk.
- Access our video stream.
- Apply the shape predictor to each individual frame.
- Verify that the results look good.
Let’s get started.
Open up
predict_eyes.pyand insert the following code:
# import the necessary packages from imutils.video import VideoStream from imutils import face_utils import argparse import imutils import time import dlib import cv2 # construct the argument parser and parse the arguments ap = argparse.ArgumentParser() ap.add_argument("-p", "--shape-predictor", required=True, help="path to facial landmark predictor") args = vars(ap.parse_args())
Lines 2-8 import necessary packages. In particular we will use
imutilsand OpenCV (
cv2) in this script. Our
VideoStreamclass will allow us to access our webcam. The
face_utilsmodule contains a helper function used to convert dlib’s landmark predictions to a NumPy array.
The only command line argument required for this script is the path to our trained facial landmark predictor,
--shape-predictor.
Let’s perform three initializations:
# initialize dlib's face detector (HOG-based) and then load our # trained shape predictor print("[INFO] loading facial landmark predictor...") detector = dlib.get_frontal_face_detector() predictor = dlib.shape_predictor(args["shape_predictor"]) # initialize the video stream and allow the cammera sensor to warmup print("[INFO] camera sensor warming up...") vs = VideoStream(src=0).start() time.sleep(2.0)
Our initializations include:
- Loading the face
detector
(Line 19). The detector allows us to find a face in an image/video prior to localizing landmarks on the face. We’ll be using dlib’s HOG + Linear SVM face detector. Alternatively, you could use Haar cascades (great for resource-constrained, embedded devices) or a more accurate deep learning face detector. - Loading the facial landmark
predictor
(Line 20). - Initializing our webcam stream (Line 24).
Now we’re ready to loop over frames from our camera:
# loop over the frames from the video stream while True: # grab the frame from the video stream, resize it to have a # maximum width of 400 pixels, and convert it to grayscale frame = vs.read() frame = imutils.resize(frame, width=400) gray = cv2.cvtColor(frame, cv2.COLOR_BGR2GRAY) # detect faces in the grayscale frame rects = detector(gray, 0)
Lines 31-33 grab a frame, resize it, and convert to grayscale.
Line 36 applies face detection using dlib’s HOG + Linear SVM algorithm.
Let’s process the faces detected in the frame by predicting and drawing facial landmarks:
# loop over the face detections for rect in rects: # convert the dlib rectangle into an OpenCV bounding box and # draw a bounding box surrounding the face (x, y, w, h) = face_utils.rect_to_bb(rect) cv2.rectangle(frame, (x, y), (x + w, y + h), (0, 255, 0), 2) # use our custom dlib shape predictor to predict the location # of our landmark coordinates, then convert the prediction to # an easily parsable NumPy array shape = predictor(gray, rect) shape = face_utils.shape_to_np(shape) # loop over the (x, y)-coordinates from our dlib shape # predictor model draw them on the image for (sX, sY) in shape: cv2.circle(frame, (sX, sY), 1, (0, 0, 255), -1)
Line 39 begins a loop over the detected faces. Inside the loop, we:
- Take dlib’s
rectangle
object and convert it to OpenCV’s standard(x, y, w, h)
bounding box ordering (Line 42). - Draw the bounding box surrounding the face (Line 43).
- Use our custom dlib shape
predictor
to predict the location of our landmarks (i.e., eyes) via Line 48. - Convert the returned coordinates to a NumPy array (Line 49).
- Loop over the predicted landmark coordinates and draw them individually as small dots on the output frame (Line 53 and 54).
If you need a refresher on drawing rectangles and solid circles, refer to my OpenCV Tutorial.
To wrap up we’ll display the result!
# show the frame cv2.imshow("Frame", frame) key = cv2.waitKey(1) & 0xFF # if the `q` key was pressed, break from the loop if key == ord("q"): break # do a bit of cleanup cv2.destroyAllWindows() vs.stop()
Lines 57 displays the frame to the screen.
If the
qkey is pressed at any point while we’re processing frames from our video stream, we’ll break and perform cleanup.
Making predictions with our dlib shape predictor
Are you ready to see our custom shape predictor in action?
If so, make sure you use the “Downloads” section of this tutorial to download the source code and pre-trained dlib shape predictor.
From there you can execute the following command:
$ python predict_eyes.py --shape-predictor eye_predictor.dat [INFO] loading facial landmark predictor... [INFO] camera sensor warming up...
As you can see, our shape predictor is both:
- Correctly localizing my eyes in the input video stream
- Running in real-time
Again, I’d like to call your attention back to the “Balancing shape predictor model speed and accuracy” section of this tutorial — our model is not predicting all of the possible 68 landmark locations on the face!
Instead, we have trained a custom dlib shape predictor that only localizes the eye regions. (i.e., our model is not trained on the other facial structures in the iBUG-300W dataset including i.e., eyebrows, nose, mouth, and jawline).
Our custom eye predictor can be used in situations where we don’t need the additional facial structures and only require the eyes, such as building an a drowsiness detector, building a virtual makeover application for eyeliner/mascara, or creating computer-assisted software to help disabled users utilize their computers.
In next week’s tutorial, I’ll show you how to tune the hyperparameters to dlib’s shape predictor to obtain optimal performance.
How do I create my own dataset for shape predictor training?
To create your own shape predictor dataset you’ll need to use dlib’s imglab tool. Covering how to create and annotate your own dataset for shape predictor training is outside the scope of this blog post. I’ll be covering it in a future tutorial here on PyImageSearch.
What’s next?
Are you interested in learning more about Computer Vision, OpenCV, and the Dlib library?
If so, you’ll want to take a look at the PyImageSearch Gurus course.
Inside PyImageSearch Gurus, you’ll find:
- An actionable, real-world course on Computer Vision, Deep Learning, and OpenCV. Each lesson in PyImageSearch Gurus is taught in the same hands-on, easy-to-understand PyImageSearch style that you know and love.
- The most comprehensive computer vision education online today. The PyImageSearch Gurus course covers 13 modules broken out into 168 lessons, with other 2,161 pages of content. You won’t find a more detailed computer vision course anywhere else online, I guarantee it.
- A community of like-minded developers, researchers, and students just like you, who are eager to learn computer vision and level-up their skills.
- Access to private course forums which I personally participate in nearly every day. These forums are a great way to get expert advice, both from me as well as the more advanced students.
To learn more about the course, and grab the course syllabus PDF, just use this link:
Summary
In this tutorial, you learned how to train your own custom dlib shape/landmark predictor.
To train our shape predictor we utilized the iBUG-300W dataset, only instead of training our model to recognize all facial structures (i.e., eyes, eyebrows, nose, mouth, and jawline), we instead trained the model to localize just the eyes.
The end result is a model that is:
- Accurate: Our shape predictor can accurately predict/localize the location of the eyes on a face.
- Small: Our eye landmark predictor is smaller than the pre-trained dlib face landmark predictor (18MB vs. 99.7MB, respectively).
- Fast: Our model is faster than dlib’s pre-trained facial landmark predictor as it predicts fewer locations (the hyperparameters to the model were also chosen to improve speed as well).
In next week’s tutorial, I’ll teach you how to systemically tune the hyperparameters to dlib’s shape predictor training procedure to balance prediction speed, model size, and localization accuracy.
To download the source code to this post (and be notified when future tutorials are published here on PyImageSearch), just enter your email address in the form below!
Downloads:
The post Training a custom dlib shape predictor appeared first on PyImageSearch.